At around 2,500 degrees Fahrenheit, steel melts. When aluminum is exposed to moisture and oxygen, it corrodes. While these conventional alloys are well suited to everyday environments, they fold under abnormally excessive exposure to extreme heat, cold, pressure and other conditions. And with the nation conducting more and more operations in extreme environments, such as space and the Arctic, the need for alloys that maintain strength under these conditions is critical.
Multi-principal element alloys (MPEAs), which are made up of several elements in roughly equal proportions, can be better suited for extreme environments because their high strength, hardness and toughness over a range of temperatures. In addition, MPEAs often exhibit excellent corrosion resistance and thermal stability, and can display unique functional properties useful for electronic or magnetic devices.
Researchers from the Johns Hopkins Applied Physics Laboratory (APL) in Laurel, Maryland, are accelerating MPEA design by creating complex microstructures that provide composition information from only a few samples. These samples, however, comprise many localized samples that are rich in data.
The team’s new pathway automatically links alloy phases—distinct materials formed when an alloy is heated or cooled—to their mechanical properties. Publishing their work in the journal Data in Brief, the researchers described a design capability they used to synthesize 17 unique MPEA compositions.
“Designing MPEAs is challenging because every small change in composition can result in large changes in material formation and properties,” said Morgan Trexler, program manager for Science of Extreme and Multifunctional Materials at APL.
“This new capability provides a path for researchers to quickly create and analyze thousands of local data within bulk samples, which will intelligently inform the design of new materials and enable rapid material discovery.”
To predict which MPEA compositions to manufacture, APL partnered with Paulette Clancy and Maitreyee Sharma Priyadarshini at the Johns Hopkins Whiting School of Engineering.
With limited data, Clancy and Sharma Priyadarshini used their physics-informed Bayesian optimization algorithm PAL 2.0 to quickly sift through alloy possibilities and recommend MPEAs that would maximize hardness and provide the widest sample of data for future alloy development.
Unlike with most deep-learning efforts, which require a large database, the team pushed to see whether few data points would still generate reasonable results. The algorithm only needed around a dozen data points to generate recommendations.
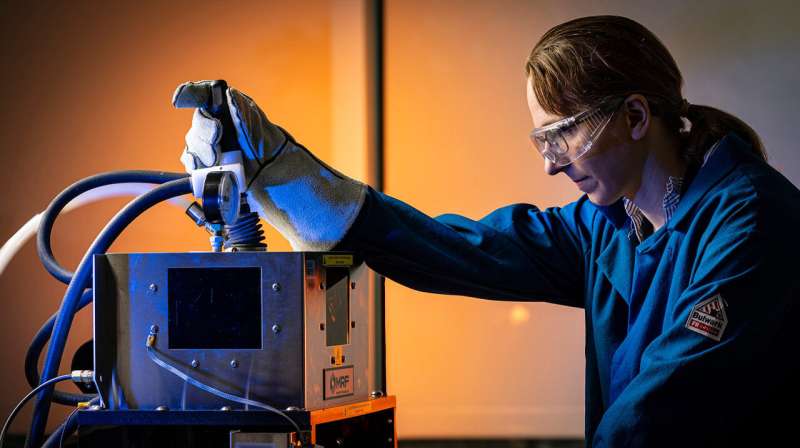
“We created a closed-loop cycle,” Clancy said. “We run APL’s data through PAL 2.0 to make predictions of compositions, APL fabricates the samples and tests the suggested alloys and analyzes the results, and then we restart the process based on the additional data collected. We’re turning the crank multiple times.”
The team continually builds off each test’s results. In the first round, the machine learning tool recommended alloy possibilities that were too expensive or unmanufacturable. The team is now in its third cycle, which is identifying alloys with more than twice the hardness.
After Clancy’s group provides recommendations on which alloys to test, the APL team creates the alloys and conducts arc melting, which sends an electronic current through the metal to melt it. Arc melting can be achieved with minimal material, making it a quick way to sample vastly different compositions.
“When the alloy is melted, it essentially creates dozens of different materials with different chemical compositions all within one sample,” said Eddie Gienger, a materials scientist at APL and lead author on the paper.
The pathway is created by integrating data from different sources: scanning electron microscopy (SEM) and energy-dispersive X-ray spectroscopy (EDS), which measure phase compositions, and nanoindentation, a process where a small triangular tip is pressed into tiny pieces of the alloy to measure hardness.
“With nanoindentation we can collect measurements from hundreds of locations automatically on the sample. We then map those indents to phase compositions identified using EDS and SEM to understand how each unique microstructure and composition will behave,” added Lisa Pogue, a materials scientist at APL.
“Identifying regions that don’t correspond to known phases enables the discovery of new, potentially useful materials. Coupling that with mechanical property information allows us to identify promising compositions for future alloy development.”
That information, which included 17 unique MPEA compositions and over 7,000 unique data points, was compiled into a database for future alloy development.
“The process helps identify the possibility space for MPEAs,” Gienger said. “If a material needs to be designed to achieve certain mechanical properties, such as hardness, the pipeline could provide the information to engineer those properties.”
The technique is one of many APL is developing and testing to speed up materials discovery and understanding. “The more tools we have to conduct high-throughput characterization, the more opportunities we have to apply it in spaces where new, stronger materials are needed,” Trexler said.
More information:
Edwin Gienger et al, A database of multi-principal element alloy phase-specific mechanical properties measured with nano-indentation, Data in Brief (2024). DOI: 10.1016/j.dib.2024.110719
Citation:
Researchers report pathway to stronger alloys for extreme environments (2024, July 16)
retrieved 16 July 2024
from https://phys.org/news/2024-07-pathway-stronger-alloys-extreme-environments.html
This document is subject to copyright. Apart from any fair dealing for the purpose of private study or research, no
part may be reproduced without the written permission. The content is provided for information purposes only.
Discussion about this post